What is Sentiment Analysis with AI? Pros and Use Cases
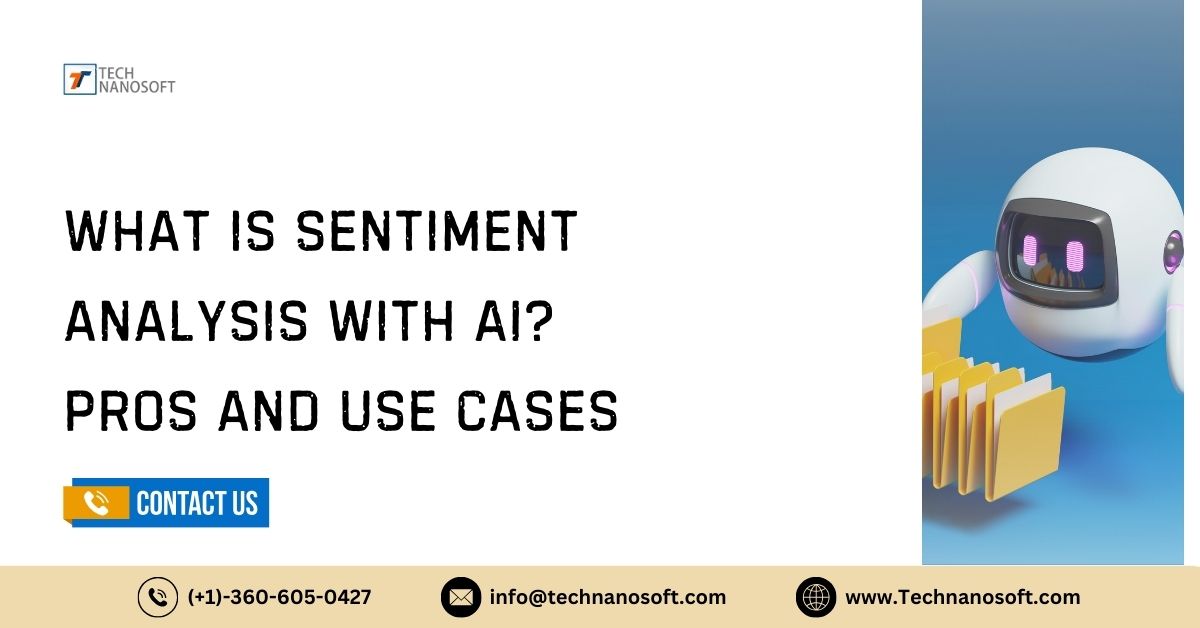
Understanding customer emotions and opinions is important for business achievements in a modern data-driven world. AI-powered sentiments analyzing provides an advanced method to analyze and categorize the emotional tone of text data. AI-driven social media and sentiment analysis, reviews, and feedback to offer essential insights into customers' views.
Businesses use such technology to improve customer satisfaction, make well-informed decisions, and strengthen their entire strategy. Understand how AI-based sentiments analysing transforms unstructured data into valuable insights contributing to additional intelligent business decisions.
What is Sentiment Analysis with AI?
Using artificial intelligence, sentiment analysis with AI recognizes and classifies a text body's emotional tone. We need to test text data to determine attitudes, views, and emotions. AI systems, such as those using NLP, can recognize emotions and context in text. They categorize the text as positive, negative, or neutral.
This technology helps businesses evaluate consumer emotions and adjust their plans accordingly. It is commonly used in market research, customer feedback analysis, and social media monitoring. The sentiment meaning to improves decision-making by offering insightful information about trends and public opinion.
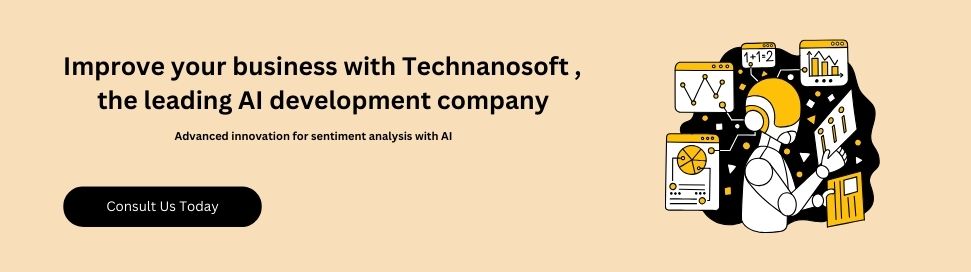
What Are Sentiment Analysis Use Cases?
Improve Customer Service
Sentiment analyzing customer emotions from social media posts, reviews, and feedback helps businesses improve customer service. Businesses may ensure customer satisfaction and trust by fixing issues discovered through early detection of negative emotions.
Analysing positive feedback helps to identify effective strategies. In addition to resolving issues, this proactive approach improves the overall quality of services. Businesses use sentiment analysis to improve customer experience and retention rates. Maintain a positive image, personalize responses to individual needs, and customize services to achieve this.
Brand Monitoring
Businesses monitor and evaluate the public views of their brand in actual time using sentiment analysis and brand monitoring. Businesses determine how people feel about their brand through social media mentions, reviews, and online discussions. This understanding helps identify problem areas and highlight effective marketing.
By maintaining things continually, businesses can immediately address negative feedback and avoid possible Publicity problems. Brand strengths can be increased by utilising positive emotions. Sentiment analysis helps shape marketing strategies and gives a clear understanding of brand health for successful communication.
Competitor And Trend Analysis
Sentiment research gives businesses insights into their industrial environment and is an effective competition and trend analyzing tool. Businesses can evaluate competitors' positive and negative opinions to determine their products' advantages and disadvantages. This approach can help in understanding the market position and comparing performance.
In addition, monitoring customer emotions and trends allows companies to plan for market changes and their strategy accordingly. Sentiment research gives businesses a competitive edge by revealing new opportunities, possible dangers, and customer preferences. This information facilitates strategic planning and well-informed decision-making.
Track Campaign Performance
Monitoring the success of advertising efforts involves the use of sentiments analysis. Businesses may track the success of their campaigns in actual time by examining consumer responses and feedback. Negative views show areas requiring work, and positive emotions show the relationship progressing.
This analysis helps in improving targeting efforts, improving content, and adjusting campaign methods. Monitoring sentiment structures over time provides valuable information on the tastes and behaviour of customers. Businesses can use sentiment analysis to ensure that their campaigns connect with their target audience. This helps them make a strong impact and achieve their desired outcomes.
Financial Market Analysis
Sentiment examines the emotional tone of financial market-related news, documents, and social media posts, an essential component of financial market analysis. Using this data, analysts and investors forecast stock price changes, assess market trends, and make accurate investment decisions.
While negative emotions suggests depressing situations, positive emotions indicate positive changes. Investors improve their awareness of market behaviour, risk management, and portfolio optimisation by including sentiment analysis in their strategies. This technology offers a thorough understanding of market actions, enhancing traditional analysis techniques.
Sentiment-Driven Tutoring
It is a technique that sentiment-driven tutoring uses to better understand students' emotional responses to educational materials. Teachers can identify categories in which students are strong or poor through feedback, answers, and transfers. This recognition makes it possible to provide immediate assistance and personalised training, which improves student engagement and performance.
Positive emotions show instructional strategies that work, while adverse emotions direct focus on areas that require improvement. Sentiment analysis helps educators understand students' emotions and needs. This understanding allows educators to be more compassionate and flexible in providing support. It helps students succeed emotionally and mentally.
Patient Feedback Analysis
Inpatient feedback analysis and sentiment analysis helps medical professionals understand patients' emotions and observations related to their therapy. Healthcare businesses can improve patient satisfaction and identify areas for improvement by evaluating reviews, surveys, and comments.
Negative emotions focus on issues that require fixing, while positive emotions show successful interactions and solutions. Better medical results, patient trust, and service quality are all helped via analysis. Sentiment analysis allows medical professionals to establish patient-centred settings, enhancing patient treatment and experience.
Early Issue Detection
Using sentiment analysis, companies identify issues early and act before they become serious. Businesses can locate increasing challenges by maintaining social media, consumer feedback, and other communication channels to identify negative emotions. Quick solutions are possible with a proactive approach, which helps minimize negative impacts on customer satisfaction and brand reputation.
Early identification helps to seamless operations, PR difficulty avoidance, and service quality maintenance. Emotion research provides valuable information for proactive approaches and allows companies to avoid issues and maintain good client relations.
READ ALSO- What is Venmo App Development Cost?
What Are The Different Types of Sentiment Analysis?
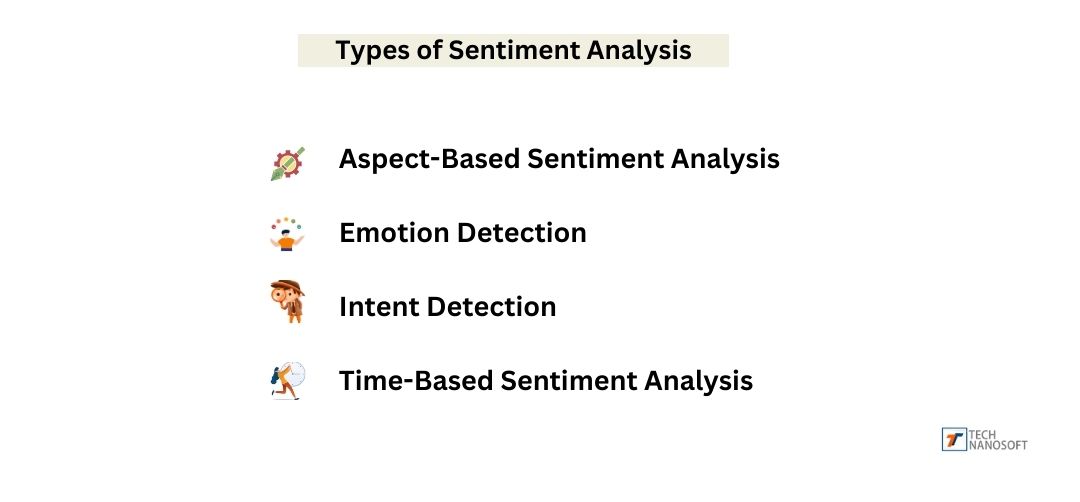
Aspect-Based Sentiment Analysis
To determine sentiment aspect-based sentiment analysis focuses on specific components or features of a product, service, or event. This approach analyses a text to evaluate each section instead of looking at the text's overall tone. For example, a restaurant evaluation conducts separate analyses for factors like environment, service, and food quality.
Businesses can make targeted modifications due to this detailed strategy, which offers better insights into clients' unique needs. Aspect-based sentiment analysis helps decipher thorough client feedback and improve specific aspects of a good or service.
Emotion Detection
Sentiment analysis operates more than simply categorizing text as positive, negative, or neutral to identify emotions. It aims to locate feelings such as happiness, pain, anger, or surprise expressed in the text. Emotion detection algorithms use advanced NLP methods to identify deeper feelings based on word choice, tone, and context.
This sentiment analysis helps learn about customers' emotions, and behaviours. Businesses can enhance customer engagement and satisfaction by using emotional strategies to better understand and respond to their customers.
Intent Detection
Intent identification is a technique of sentiment analysis focused on understanding the text's purpose or meaning. The sentiment analysis process analyses the planned action or results the author or speaker seeks to achieve. It applies to making an issue, requesting support, finishing a purchase, or collecting data.
Businesses can meet customer demands and improve the user experience, and they can accurately discern intention. It involves customer service, chatbots, and virtual assistants, where understanding the consumer's objective is essential for accurately providing helpful assistance and intent beneficial monitoring.
Time-Based Sentiment Analysis
Examining how sentiment evolves with time is the primary objective of time-based sentiment analysis. Businesses can identify trends and market changes by tracking sentiment trends across various periods. This analysis helps monitor events, marketing efforts, or new product introductions influencing consumer perception.
Making data-driven judgments and understanding the long-term effects of business decisions is made more accessible. Time-based sentiment analysis helps businesses understand how emotions evolve. This allows them to adapt their strategies accordingly. By staying informed on customer needs and industry trends, businesses can remain competitive.
How Does Sentiment Analysis Work?
In sentiment analysis, natural language processing (NLP) and machine learning methods used to examine text data. The system filters and tokenizes the text as part of the preparation step. After that, we examine the text to find context and subjective information. Algorithms categorise the text into groups like beneficial, negative, and neutral.
More advanced algorithms may also identify particular feelings or objectives. Over time, the algorithm improves and becomes more accurate according to training data. Emotion analysis is used to analyse data from various sources, including social media, reviews, and customer feedback. This process provides firms with helpful information about public sentiment and facilitates decision-making.
READ ALSO- How Ai work in Softawre Development and How to Make an Ai?
What Are The Approaches To Sentiment Analysis?
Rule-Based Approach
It define sentiment collection of linguistic rules is applied in the rule-based method to sentiment analysis to assess and classify text. These guidelines typically address grammar and syntactic patterns and use dictionaries that contain both positive and negative words. The system determines the sentiment expressed in the text by using these rules.
While this method is easy to grasp and use, it may not be as precise or adaptable as more advanced methods. When domain-specific rules are clear, rule-based techniques serve as a solid foundation for sentiment analysis systems and are helpful for straightforward applications.
Lexicon-Based Approach
A sentiment lexicon is a list of words and phrases with assigned sentiment scores, used for sentiment analysis. Every word in the lexicon has a emotion value capable of being positive, negative, or neutral. To determine the overall emotion, the method compares terms in the text to the lexicon and then combines the ratings of words.
By changing the lexicon, this technique becomes tailored to domain-specific needs and is efficient for managing large volumes of data. Lexicon-based analysis struggles with understanding context and complex phrases. However, it provides a good balance between simplicity and accuracy when compared to machine learning.
Machine Learning Approach
Text sentiment definitioned using algorithms and models with large datasets through machine learning for sentiment analysis. A model is developed based on identified information, meaning every text example's emotion. Naive Bayes, Support Vector Machines, and deep learning models like neural networks include standard algorithms. With new unseen text, the trained machine detects similarities and forecasts emotions.
Machine learning technologies offer superior accuracy and flexibility for managing complex linguistic nuances and context than rule-based or lexicon-based approaches. They offer reliable and scalable sentiment analysis solutions but also have a high data and processing resource requirement.
Hybrid Approach
The hybrid Approach for sentiment analysis combines lexicon-based, rule-based, and machine-learning techniques. Each technique leverages its unique strengths. This method starts through processing and filtering text using lexicon- and rule-based techniques to obtain initial sentiment analysis. This method starts through processing and filtering text using lexicon- and rule-based techniques to obtain initial sentiment analyzed.
Subsequently, these ratings are improved using machine learning models, which analyse vast datasets to identify trends and context. The hybrid method addresses individual techniques' drawbacks, which increases accuracy and flexibility.
This tool is great for analyzing emotions in various contexts. It excels at recognizing sarcasm, complex language, and diverse expressions. This makes it a valuable resource for understanding the nuances of human communication.
How Does Technanosoft Help with AI Sentiment Analysis?
Technanosoft offers customized AI solutions to meet various business needs, improving sentiment analysis. Technanosoft uses machine learning and NLP to analyze client feedback from sources like social media, reviews, and surveys accurately.
This complete strategy includes real-time monitoring, thorough sentiment classifying, and valuable information. It makes it achievable for businesses to make data-driven decisions, improve customer satisfaction, and comprehend customer attitude. With Technanosoft's experience, sentiment analyzing is correct, which benefits businesses by enhancing customer engagement, brand reputation, and overall market strategy.
FAQs
Q.1- What is a sentiment classifier?
A- An AI-powered sentiment classifier evaluates and classifies text data based on emotional tones. NLP and machine learning are used to determine the sentiment of a text by identifying the emotion it conveys. This process categorizes the text as positive, neutral, or negative based on the identified emotion. The goal is to understand the overall tone or feeling conveyed in the text.
Businesses can understand public opinion and emotional responses via sentiment classifiers, which process data from social media, reviews, and customer feedback. These tools help businesses improve their products, services, and general customer experience by recognising subtle emotions and offering insightful data on consumer behaviour.
Q.2- What does sentiment analysis do?
A- Sentiment analysis, also called opinion mining, analyses and categorises the emotional tone of text data using artificial intelligence (AI) and natural language processing (NLP). It determines the positive, negative, or neutral sentiment by examining user reviews, social media posts, and other text sources. Businesses gain significant knowledge of consumer emotions using this method, which helps understand customer happiness, improve goods and services, and make decisions. By addressing problems and utilising favourable trends, sentiment analysis allows businesses to maintain an excellent image, improve customer experience, and react quickly to feedback.
Q.3- What is sentiment analysis in machine learning?
A- In machine learning, sentiment analysis provides algorithms to categorise text according to its emotional aspects. These algorithms learn to identify sentiment-indicating features and related information from datasets with labels. Neural networks, Support Vector Machines, and Naive Bayes include standard models. The models can accurately predict the emotion of fresh, unread material after being trained.
This method successfully handles intricate linguistic intricacies while providing excellent accuracy and flexibility. Businesses can measure consumer emotions, forecast trends, and make data-driven decisions with machine learning-based sentiment analysis. It improves overall strategy and customer happiness, providing firms with solid tools.
Q.4- What is NLP sentiment analysis?
A- Sentiment analysis in nlp serve to evaluate and categorise textual data's sentiment in NLP sentiment analysis. Positive, harmful, or neutral emotion identifies NLP algorithms by understanding language's structure, meanin g, and context.
This approach prepares the text, collects features, and applies machine learning models for sentiment analysis. NLP sentiment analysis is frequently employed to examine comments, social media interactions, and consumer evaluations. Businesses improve their products, services, and customer experiences by utilising actual time emotional data that provides deep insights into customer attitudes.